Major progress in the theoretical description of the internal structure of the proton and neutron over the last 15-20 years has led to breakthroughs in our understanding of the mechanisms by which their components, the fundamental quarks and gluons, constitute observable matter. In concert with these theoretical realizations, advances in particle-accelerator and experimental-detection technologies, along with dramatic developments in leadership-class computing capabilities and artificial intelligence, such as machine learning and deep learning, have brought us to the threshold of the new science of nuclear femtography. Nuclear femtography will enable a three-dimensional picture of the interior of the proton to emerge.
The challenges posed by leveraging these advances are formidable and exciting. Large amounts of data will need to be analyzed. This analysis will involve reconstruction of physical quantities from the raw data and integration of diverse datasets from experiments across the globe into physical observables that encapsulate the information describing the three-dimensional distributions of quarks inside protons and neutrons.
The production of experimentally determined distribution functions, and their calculation from first-principles theoretical computation, will require leading-edge computation. Machine-learning methods will play a major role in understanding the correlations within the data, in revealing the optimal distributions, and in identifying the importance and impact of future experiments.
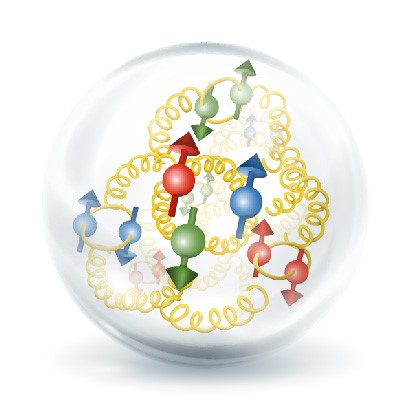
MISSION
The mission of the Center for Nuclear Femtography is to coalesce the expertise in nuclear physics, computational science, mathematics and visualization to establish the world-leading center for three-dimensional imaging of nucleons and nuclei. The center will thereby train the next generation of scientists and technologists, educate students across Virginia, and advance the role of the Commonwealth as a focus for emerging technologies.
COLLABORATIONS
Quark Gluon Tomography Collaboration
FUNDING
The Center for Nuclear Femtography is funded by the Southeastern Universities Research Association (SURA) under an appropriation from the Commonwealth of Virginia.